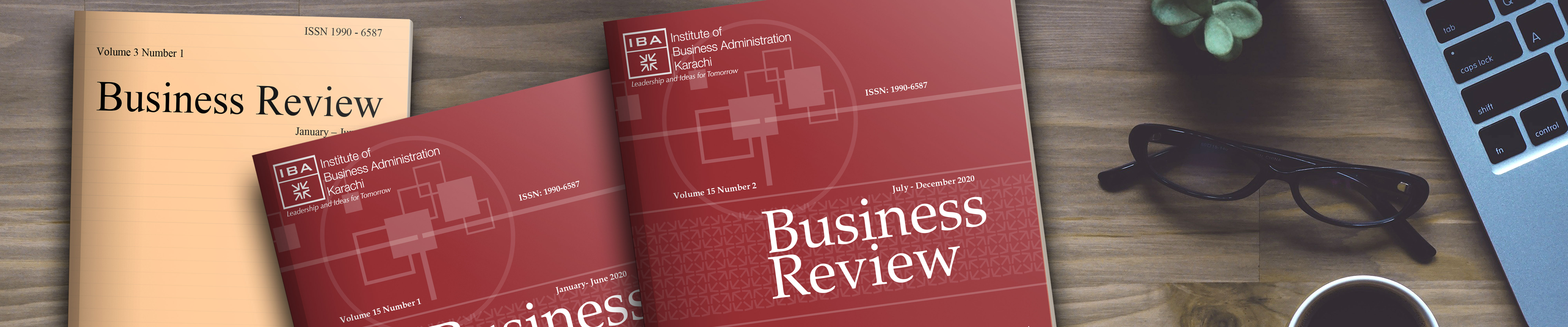
Author ORCID Identifier
Rabia Sabri ORCID: 0000-0002-9135-2137
Abstract
This research is focused on the stock exchanges of Sri Lanka, Pakistan, and Bangladesh due to their significant presence in the Asian region and the unique challenges, opportunities, and presence in these frontier markets. The study assesses both traditional stationary models, Autoregressive Integrated Moving Average (ARIMA) and Theta traditional stationary models, with the contemporary deep learning models Long Short-Term Memory (LSTM) with 1D Convolutional Neural Network (CNN) support. The study covers the sample period of historical data from 2020–2023 to include the in and out of sample forecasting of 2024–2025. The time series comprises linear and nonlinear datasets to capture a wider range of factors influencing the market. The evaluation metrics are used to balance the prediction model's accuracy and the intricate dynamics of the markets. The conventional time series models hold the advantages of their interpretability and computational efficiency, but the combined effect of CNN-LSTM exhibits significantly superior accuracy in predictions. Integrating advanced techniques with traditional statistical methods provides a more comprehensive and accurate forecast to capture the complex Intricacies of stock indices. The ensembling model approach can improve predictive performance and help stabilize the market ecosystem.
Keywords
Stock Market forecasting, Frontier market, univariate, multivariate, ARIMA, Theta Deep learning models, Ensemble Learning Techniques
DOI
10.54784/1990-6587.1656
Journal of Economic Literature Subject Codes
C32, C45, C52, C53, D53, G15, G17
Creative Commons License
This work is licensed under a Creative Commons Attribution 4.0 International License.
Recommended Citation
Sabri, R., & Iqbal, S. (2024). Comparative Analysis of Univariate and Deep Learning Models for Stock Market Prediction in Frontier Markets: A Case Study of Pakistan, Bangladesh, and Sri Lanka. Business Review, 19(2), 54-73. Retrieved from 10.54784/1990-6587.1656
Submitted
May 28, 2024
Revised
October 18, 2024
Accepted
November 01, 2024
Published
November 11, 2024
Included in
Publication Stage
Online First